“History doesn’t repeat itself but it often rhymes” – Mark Twain
“You can’t beat the market” is a popular phrase that a lot of people believe in. It comes from the famous ‘Efficient Market Hypothesis’ according to which, the price of any and every financial asset is a completely optimal and justified estimate of its investment value, reflecting all the information available. The future price estimates will follow a random walk and thus an investor is unlikely to beat the market. This theory underpins other modern risk based theories, like Capital Asset Pricing Model and concepts like beta. However, if no one can really beat the market how do investors such as Warren Buffet and many others make huge profits and how does it happen almost continuously, it can’t be a mere happenstance all the time. That leads us into believing that we could possibly predict the stock prices, unlike what the Efficient Market Hypothesis propounded. Well, the answer to that might actually be yes and no!
We live in an interconnected society and in today’s world what happens in Vegas doesn’t really stay in Vegas. Organisations and markets behave like networks that have chaotic and complex systems and the world of science has been mostly confined to linear systems and its workings, overlooking the range of possibilities in a dynamic and unpredictable system. The Chaos theory is one such interesting theory that attempts to explain the non-linear and dynamic systems. In an attempt to define this theory Edward Lorenz had said, “Chaos: when present determines the future, but the approximate present doesn’t approximately determine the future”. Such complex chaotic systems are vulnerable to slight changes in its initial conditions that can cause big shifts in its results, this is termed as the ‘Butterfly effect’. Benjamin Franklin once offered a poetic perspective in his variation of a proverb that’ll better explain this effect:
“For the want of a nail the shoe was lost,
For the want of a shoe the horse was lost,
For the want of a horse the rider was lost,
For the want of a rider the battle was lost,
For the want of a battle the kingdom was lost,
And all for the want of a horseshoe nail.”
The lack of a horseshoe nail sounds as unimportant but indirectly caused the loss of a war, similarly a tiny initial nudge may in itself be inconsequential but one never knows what huge effect it can have on the final outcomes. This effect can be applied to all open systems, that is all systems wherein new factors can be added and subtracted as time goes by, in stock markets there could be thousands of initial points as there are thousands of investors who enter and exit and carry out transactions at the same time. This theory can even be applied to the changes in the weather, the system of which is indeed very comparable to the behaviour of financial markets. Coming back to chaotic systems, we usually have two basic types of feedback loops, the positive and the negative feedback loops. The positive feedback loop tends to increase the effect of initial variables, moving out of equilibrium and sometimes exponentially, that can lead to the failure of a system. The negative feedback loop in contrast, tends to bring about stability by going in the opposite direction of the initial change. When both these mechanisms work simultaneously that causes a dynamic equilibrium and is what probably happens in the stock market, where the prices keep oscillating with varying degrees.
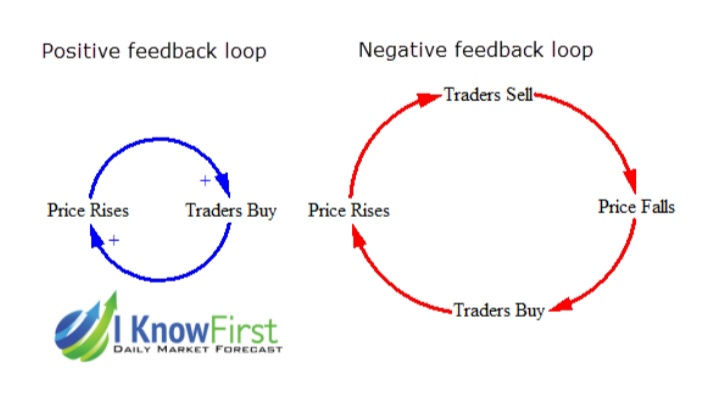
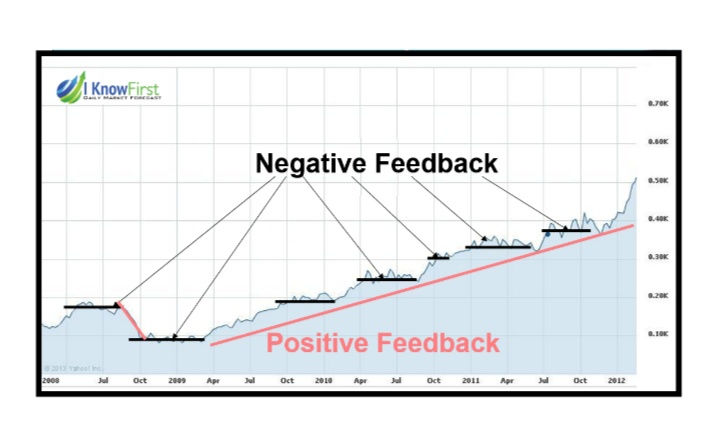
One more interesting thing to note is that these systems behave predictably for a while and then may shift gears without warning, to enter into another mode of behaviour that’ll be different but predictable. Shifts like that can occur many number of times and could be predictable every time but with a different set of rules. This is shown by the Lorenz attractors, how important the sensitive dependence on initial conditions can be, this is true for the behaviour exhibited in the stock market. We have the bull and bear markets to which investment strategies and reactions are different, so when people provide varying initial inputs in both these types of markets, the outcomes will ultimately get affected and both will lead to different results.
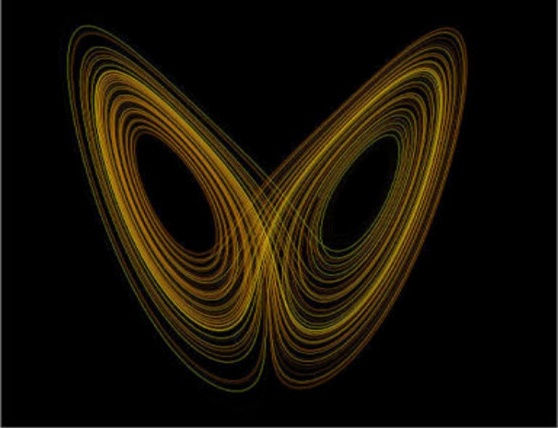
A plot of the Lorenz attractor(for specific values), looks like a ‘butterfly’ doesn’t it?
Chaos theory attempts to look less at details and more at the overall patterns of change, and that is how the financial markets are studied and the trends are made. In 1991, a group of men including Doyne Farmer, Norman Packard and James McGill had started the Prediction Company that employed Chaos theory into their predictions of market movement with much success. Despite market prices being random they still have some kind of a trend, as Packard said that the success they got was by recognizing “pockets of predictability” in a sea of other complex systems that often mask these simpler shorter “familiar swirls” in the stock market. If one can identify them then it is possible to take advantages of high returns on investments. One more concept in Chaos theory is of the fractals that can again be applied to the market. As defined by Mandelbrot “A fractal is a geometric shape that can be separated into parts, each of which is a reduced-scale version of the whole”. The stock market seems to have similar characteristics because at closer time periods the prices tend to generate more and more detail. In other words, if you zoom in or out over a historical price plot of the stock market so that it fits the same time and price scale, you’ll not be able to tell which graph shows the weekly, daily and hourly changes, because they all look more or less similar due to the detail.
From the above, the Fractal market hypothesis can be considered to be a better theory than the Efficient market hypothesis. According to the former, the market comprises of many investors with different investment horizons, which means the investment time for all investors could vary and so the price changes are due to information being meaningful only to a certain investment segment. Therefore equilibrium price does not exist because people value investments differently. This hypothesis as, opposed to the earlier one also allows for major and unlikely events to happen like the stock market crashes and bursting of economic bubbles, which is what happens in reality. However, there is no best fit theory for the stock market, though the Fractal market hypothesis claims to predict the ‘cyclical’ and ‘repetitive’ pattern of the stock market in the same way as fractals tend to repeat themselves along all time frames. The main problem with this is deciding the length of time in which a fractal pattern should repeat itself in a market scenario. Also, it is not really possible to include every variable in the market into the hypothesis, and more the variables more closely will the replication to the real market conditions and accuracy be. Moreover, it is understood that most complex systems have both a systematic and a random component, and that can only allow us to forecast stock market realistically to a certain extent and not beyond. Rules we infer from the observation of events can never really contain the full range of possibilities (‘The Black Swan’, Nassim Taleb). So, even if history may not exactly repeat itself, in the words of Tom Konrad, isn’t knowing the limits of our ability to predict more important than the predictions themselves?
- Pamona Sharma
References-
Comments